Overview
When interacting with a robot, humans form conceptual models (of varying quality) which capture how the robot behaves. These conceptual models form just from watching or interacting with the robot, with or without conscious thought. Some methods select and present robot behaviors to improve human conceptual model formation; nonetheless, these methods and HRI more broadly have not yet consulted cognitive theories of human concept learning. These validated theories offer concrete design guidance to support humans in developing conceptual models more quickly, accurately, and flexibly. Specifically, Analogical Transfer Theory and the Variation Theory of Learning have been successfully deployed in other fields, and offer new insights for the HRI community about the selection and presentation of robot behaviors. Using these theories, we review and contextualize 35 prior works in human-robot teaching and learning, and we assess how these works incorporate or omit the design implications of these theories. From this review, we identify new opportunities for algorithms and interfaces to help humans more easily learn conceptual models of robot behaviors, which in turn can help humans become more effective robot teachers and collaborators.
What is Human Concept Learning?
Cognitive theories of human concept learning explain how humans come to understand complex, high-dimensional phenomena and make predictions about unrevealed facts and futures—as such, these theories can be applied to help humans understand how robots act in the world. We look to two complementary theories, Analogical Transfer Theory and the Variation Theory of Learning, to inform how interfaces can best mediate the practice of humans learning about robot behaviors.
Variation Theory supports humans in distinguishing core knowledge from superficial or incidental details, and Analogical Transfer Theory supports humans in transferring knowledge to new concepts or phenomena. Variation Theory prescribes an ordered sequence of variance and invariance to help humans distinguish core behaviors from superficial or incidental details, while Analogical Transfer Theory prescribes knowledge transfer by aligning new and unknown concepts with familiar entities. Together, these theories provide design guidance for human-robot interaction interfaces and algorithms, i.e., about the selection, sequence, and presentation of robot behaviors.
Human Concept Learning Principles in HRI Papers
We examine how principles of human concept learning have been used in past efforts to build HRI systems.
Paper
PDF of the paper: Available Here
Citation
bibtex
@misc{BoothHRI2022,
title={Revisiting Human-Robot Teaching and Learning Through the Lens of Human Concept Learning},
author={Serena Booth and Sanjana Sharma and Sarah Chung and Julie Shah and Elena L. Glassman},
year={2022},
journal={ACM/IEEE International Conference on Human-Robot Interaction (HRI)}
}
Contact Details
Co-author contact details are available in the paper! Download the PDF above to view.
Acknowledgements
Website adapted from MiniConf by Hendrik Strobelt and Sasha Rush.
This work was supported in part by NSF Grant No. 2107391. Serena Booth is supported by an NSF GRFP.
Authors
Sanjana Sharma
Harvard Graduate School of Design
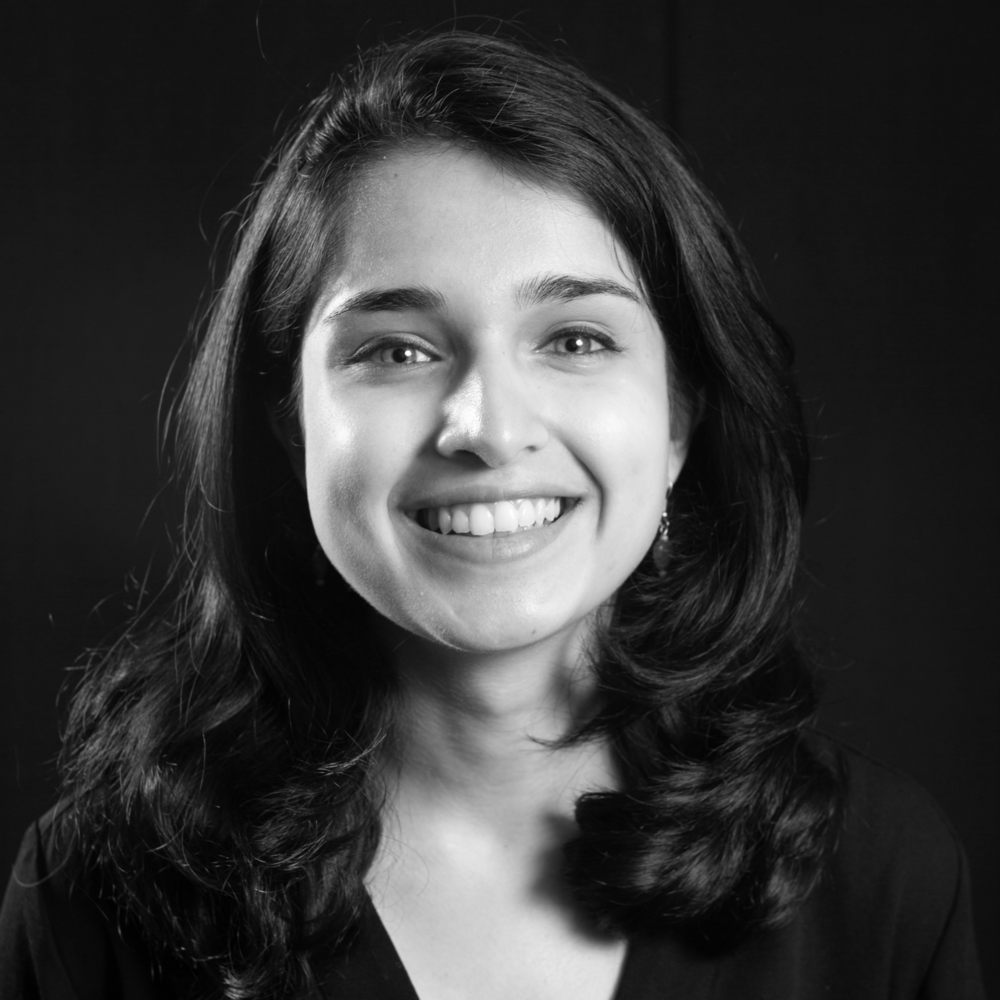
Sarah Chung
MIT CSAIL, Harvard SEAS
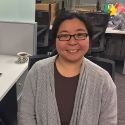